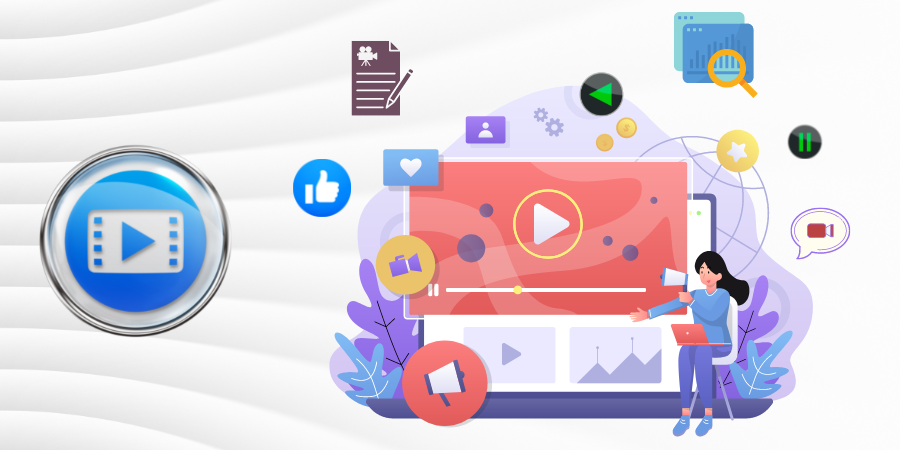
In today's digital world, numerous videos are generated and distributed through various forms of media. Since these videos are uploaded to the internet or cloud, browsing and managing video data demands high-speed computing technology. To overcome these restrictions, video summarization emerged as a savior.
Video summarization provides a compact representation of an actual video clip by displaying the most representative synopsis of a given video sequence.
This article details the definition, application, and techniques of video summarization.
Definition of Video Summarization
Video summarization is producing a summary of the content of a lengthier video by selecting and presenting the most informative or intriguing materials to potential users. The output summary comprises a collection of video clips taken from the original video with some editing processes.
The produced summary is often made up of a collection of representative video frames (or video key-frames, or video storyboard) or video fragments (or video key-fragments, or video skim) stitched together in chronological order to make a shorter video.
In video summarization, the input is a video with original content; it aims to accelerate browsing a huge cluster of video data while establishing efficient access to the video content. Based on the application and intended users, the summary evaluation is conducted to assess a summary's content, informativeness, and quality. And by reviewing the synopsis, users can make quick decisions about the video's worthiness.
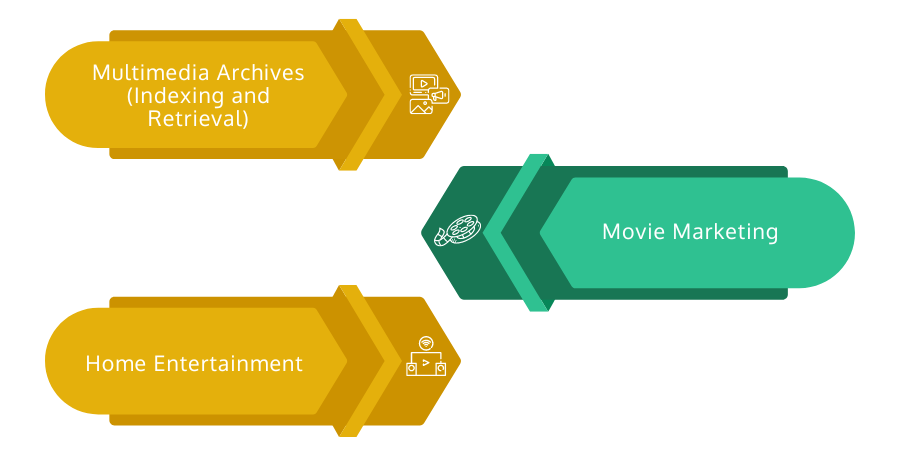
Applications of Video Summarization
Video summarization can be used in many applications.
Multimedia Archives (Indexing and Retrieval)
Over the years, many video documents have been digitized and archived. Video summarization is used to index and retrieve a vast video collection while making video content more accessible.
For example, online summarization helps journalists search for outdated video content or to make documentaries. Another example is the widespread usage of web videos, where the index can be achieved with automatedly generated video summaries.
Movie Marketing
In the broadcasting and filmmaking industries, a large amount of raw footage is used for making end products such as TV shows and movies. With multiple instances, video footage is usually shot up twenty to forty times before becoming the final-finished product.
As the "shoot-to-show" ratio fluctuates between 20 and 40, the producers consider these massive raw videos a low-cost bonanza. Therefore, video summarization identifies the stock footage from inessential materials for effective reuse.
Home Entertainment
Video summarization, for example, is used to obtain a quick summary of what happened in a television series that might remain unnoticed.
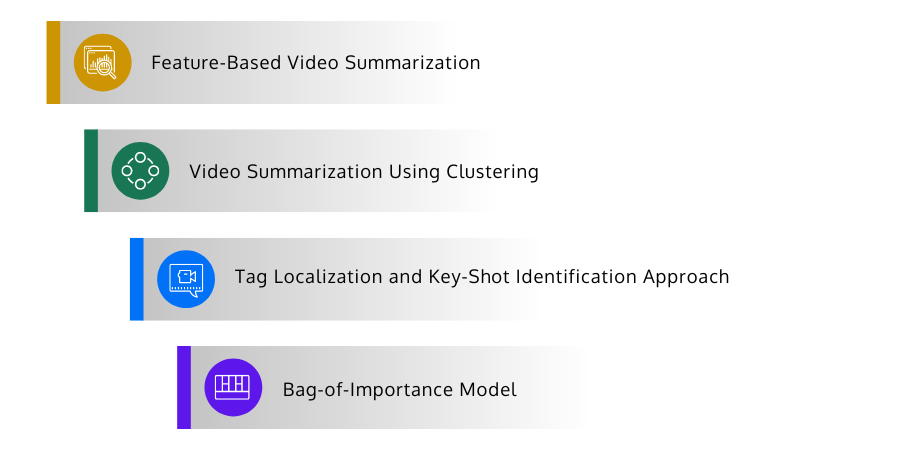
4 Video Summarization Techniques
Feature-Based Video Summarization
Digital videos comprise color, action, voice, and other characteristics. This technique is effective when users want to concentrate on the video's features. For example, if a user observes color features, color-based video summary techniques should be used. Similarly, video summarization techniques are categorized based on motion, color, dynamic contents, gesture, audio-visual, voice transcripts, and objects.Video Summarization Using Clustering
Clustering is the most widely used technique when users face similar activities or qualities within a frame. This also helps to remove frames with irregular rends. While other video summarization methods allow more efficient video browsing, they also provide video summaries- either too long or need to be clarified.
Clustering-based video summarization is categorized into similar segments, for example, K-means clustering, partitioned clustering, and spectral clustering, to define features of any video.
Tag Localization and Key-Shot Identification Approach
Tag localization is accomplished using a bag-of-instances model. Several videos are available online with rich metadata, such as titles, comments, and tags. A new effort has been put into action to search or explore the tag information of any web video. A particular system enriches YouTube videos' tag information by identifying redundancy, overlapping, or repetitive content. They develop a graph for a series of videos, and tags can be transferred to the target video.
Key-shot identification is performed using the assumption that videos appear several times in search results. This type of identification can be achieved by near-duplicate detection, which involves performing near-duplicate detection using the keyframe pairs taken from various web videos. As near-duplicate keyframes hold minor differences, the clustering-based technique accelerates the key-shot identification detection process.
Bag-of-Importance Model
A video is a collection of weighted features. The representative frame can be recognized by combining the weighted features. The Bag-of-importance model offers an approach to utilize both inter-frame and intra-frame properties by quantifying the relevance of individual features. A video sequence is projected into a low-dimensional sparse space.
In the End
Video summarization is a powerful approach for video browsing and accessing. A brief and informative video summary allows users to quickly determine the overview of video content and choose whether the entire video is worth viewing. A good understanding of semantic video content is required to make video summarization extremely scalable, reliable, and efficient. In the near future, many breakthroughs will be made to build and optimize the best video summaries based on the audience, delivery channel, and summarising intent.