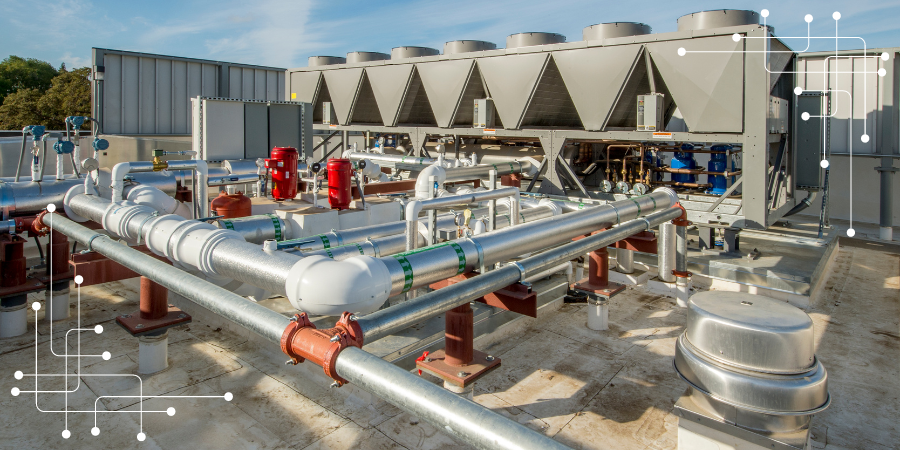
Introduction
The HVAC (Heating, Ventilation, and Air Conditioning) industry is crucial in maintaining indoor comfort and air quality. With the advent of AI (Artificial Intelligence), there is a significant opportunity to enhance climate optimization, resulting in improved energy efficiency, better occupant comfort, and reduced operational costs. This blog outlines a comprehensive approach to deploying AI for in-house climate optimization in the HVAC industry, detailing each phase and component.
Batoi Approach
In deploying AI for HVAC climate optimization, Batoi follows a systematic and comprehensive approach. This approach ensures that each phase, from data collection to continuous improvement, is meticulously planned and executed to achieve optimal results. Below is a detailed overview of Batoi's methodology:
1. Data Collection and Integration
a. IoT Sensors Deployment
To begin, IoT sensors should be installed throughout the buildings to monitor various parameters such as temperature, humidity, air quality, and occupancy. These sensors are the foundation for data-driven climate control.
b. Data Aggregation
The data collected from these sensors must be aggregated into a centralized cloud platform. This platform should be capable of ingesting and storing real-time data, ensuring that all relevant information is available for analysis.
2. Data Preprocessing
a. Data Cleaning
AI algorithms can clean the collected data by removing noise and handling missing values. Clean data is essential for accurate analysis and model training.
b. Normalization and Standardization
Normalization and standardization processes ensure the data is consistent and ready for further analysis. This step is crucial for maintaining the integrity of the predictive models.
3. AI Models for Predictive Analytics
a. Predictive Maintenance
AI models can be developed to predict equipment failures proactively. This allows for scheduled maintenance, reducing downtime, and enhancing the reliability of HVAC systems.
b. Energy Consumption Forecasting
Using historical data, models can be built to forecast future energy consumption patterns. This helps optimize energy usage and reduce costs.
c. Occupancy Prediction
AI models can predict building occupancy levels at different times of the day, enabling dynamic adjustment of HVAC settings to match occupancy patterns.
4. Real-Time Climate Control
a. Adaptive Control Systems
Reinforcement learning algorithms can be deployed to create adaptive control systems. These systems learn the optimal HVAC settings based on real-time data and environmental conditions.
b. Feedback Loops
Establishing feedback loops allows the system to continuously monitor the indoor climate and adjust HVAC operations to maintain optimal conditions.
5. Advanced Analytics for Climate Optimization
a. Anomaly Detection
AI algorithms can detect anomalies in climate conditions or sensor data, enabling timely interventions to prevent overheating or poor air quality.
b. Optimization Algorithms
Optimization algorithms can balance energy consumption and climate comfort efficiently, considering variables such as weather forecasts and energy prices.
6. User Interface and Reporting
a. Dashboard Development
Developing intuitive dashboards allows building managers to monitor real-time climate conditions, energy usage, and system performance.
b. Automated Reports
Automated reports provide insights and recommendations based on data analytics, helping decision-makers optimize climate settings further.
7. Scalability and Continuous Improvement
a. Scalable Architecture
Ensuring that the cloud platform and AI models are scalable is crucial to handle increasing data as more sensors and buildings are added.
b. Continuous Learning
Implementing a continuous learning mechanism allows AI models to be regularly updated with new data, improving their accuracy and performance over time.
Deployment Scenario
PHASE | ITEM | ACTION PLAN |
---|---|---|
Initial Phase | Pilot Program | Start with a pilot program in a selected building or area to test the AI/ML systems and gather initial data. |
Sensor Installation | Install IoT sensors in strategic locations within the building to collect comprehensive data. | |
Model Training | Train initial AI/ML models using historical and real-time data from the pilot program. | |
Expansion Phase | Scaling Up | Gradually expand the deployment to additional buildings based on insights and performance from the pilot program. |
Model Refinement | Continuously refine and improve AI models with new data from expanded deployments. | |
Integration with Other Systems | Integrate AI/ML systems with other building management systems (BMS) for a holistic approach to climate control. | |
Long-Term Phase | Fully Autonomous Operation | Aim for a fully autonomous HVAC control system that self-optimizes based on real-time data and predictive analytics. |
Advanced Features | Introduce advanced features such as voice control, mobile app interfaces, and personalized climate settings for occupants. | |
Global Optimization | Leverage cloud-based analytics to optimize HVAC operations at the building level and across multiple buildings in a portfolio. |
Use Cases
1. Energy Savings
AI algorithms can optimize HVAC settings to reduce energy consumption by predicting periods of low occupancy and adjusting the system accordingly. For example, when a building is expected to be less occupied, such as late at night or during holidays, the AI system can lower heating or cooling efforts to save energy. Additionally, by analyzing historical data and weather forecasts, AI can adjust HVAC operations in advance to ensure energy is used efficiently. This reduces energy bills and contributes to environmental sustainability by lowering carbon footprints.
2. Comfort Improvement
AI models can learn occupant preferences and adjust climate settings to enhance comfort while maintaining energy efficiency. By gathering data on individual preferences and typical usage patterns, the AI can create personalized climate zones within the building. For instance, if different individuals or groups consistently use certain rooms, the system can adjust temperatures and humidity levels to match their comfort needs. Moreover, the AI can adapt to changing preferences over time, ensuring that the indoor environment remains comfortable for all occupants without requiring manual adjustments.
3. Preventive Maintenance
Predictive maintenance models can forecast equipment failures, allowing for timely maintenance and reducing unexpected downtime. AI can analyze data from various sensors and detect early signs of wear and tear in HVAC components, such as unusual vibrations or temperature fluctuations. Maintenance can be scheduled conveniently by predicting when a component will likely fail, preventing unexpected breakdowns that could disrupt operations. This approach extends the lifespan of HVAC equipment and reduces maintenance costs by avoiding emergency repairs and optimizing the use of maintenance resources.
4. Air Quality Management
AI can monitor air quality in real-time and adjust ventilation to ensure healthy indoor environments. By continuously analyzing data on pollutants, CO2 levels, humidity, and other air quality indicators, the AI system can make real-time adjustments to ventilation rates, filtration, and air circulation. For instance, the AI can increase ventilation to maintain air quality standards during high outdoor pollution or indoor activity levels that generate more pollutants. This is particularly important in offices, schools, and hospitals where maintaining healthy air quality is crucial for occupant health and productivity.
Conclusion
Integrating AI in HVAC systems offers significant benefits, including enhanced energy efficiency, improved occupant comfort, and reduced operational costs. By following a structured deployment approach, HVAC systems can become more adaptive, predictive, and efficient, paving the way for smarter, greener buildings.